The last 10 years or so has seen a huge increase in the amount of data being collected. More and more advanced metrics are breaking into the ‘mainstream’. Clubs are investing in data analysts and even setting up whole departments to parse through this data looking for anything that will give them an advantage.
While there’s always a person, a club or a department trying something new - football, as an industry, is notoriously conservative and resistant to change. But, when a change is inevitable, no one wants to be left behind!
Hadi Sotudeh, a doctoral student in football analytics at ETH Zürich - one of the world's top universities - is at the forefront of this shift. Specializing in the use of tracking data to analyze tactics and formations, Hadi has gained recognition within the football data community, particularly on LinkedIn, where he regularly shares job opportunities in the field.
The interview has been condensed and lightly edited for grammar and clarity.
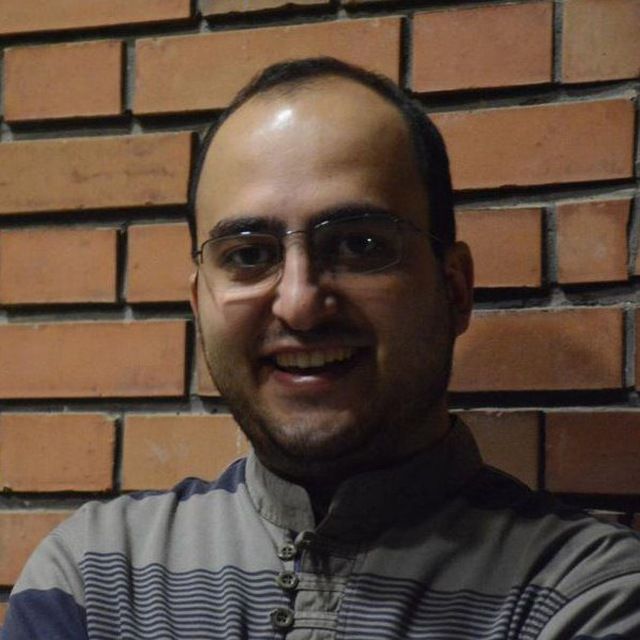
[ Background ]
I was always watching football and also playing with friends from time to time. But I'm coming from a computer science and software engineering background from my bachelor's back in Iran. After that, I did a master's in data science in the Netherlands and Sweden (a double degree program in partnership with the European Institute of Innovation and Technology). Mainly I was taking general courses in data science, machine learning, data mining, and process mining. The idea was to learn the methods that one can use to analyze data, no matter the domain.
After my master's studies, I went back to the Netherlands and started a program called ‘Engineering in Doctorate (EngD)’ in data science. It was a two-year program where you’re employed by the university and work on projects with the university's partners.
I thought, ‘Okay’, this program will end in 2 years, what do I want to do after that?’ As a data scientist, there are many different industries that you can work in. I thought about which industry would keep me motivated to spend more than a year in because I didn’t want to be the person who moves between different industries frequently. I didn’t have a clue how data was being employed in football but I saw some people were data scientists at football clubs. I decided to make the most out of the free time I had and learn what others were doing in the football industry.
That was around 2018, I started from zero and just started contacting people who worked as data scientists on LinkedIn. I asked for resources and articles I should go through to understand the domain. I think it’s really important as a successful data scientist that in addition to the techniques, you should know about the field you’re working in.
I thought because I watched football and knew something about it, it was going to be easier for me to get into it. Maybe that’s the impression a lot of people have that more or less follow football - probably you know more about football than e.g. the banking sector, so it’s easier. As you go forward though, there are a lot of hidden aspects - different terminology, tactics, and applications of data. Football clubs, like any other business, want to use data to reduce costs and increase revenue!
In the 1st year of the Engineering Doctorate program in the Netherlands, Paris Saint-Germain organized a sports analytics competition. I attended with ~3,000 other participants and made it to the final round. I went to Paris and gave my presentation there at a conference. In the end, I finished 3rd in that competition, I think this really helped me. For example, after this, I started my one-year thesis for the Dutch Football Association. After graduation, I continued with them as a part-time freelancer. Three years ago, I found an opening for a PhD position on soccer analytics and applied! Now I’m in my 3rd year.
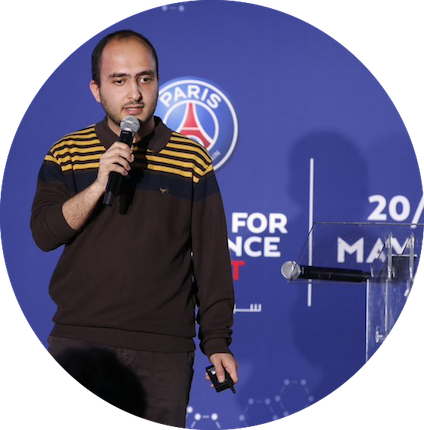
[ What is the subject of your PhD? ]
I am in the 3rd year of my doctoral studies at ETH Zürich where I’m mainly focusing on studying team tactical formations from tracking data. So, 4-4-2, 3-5-2, 4-2-3-1, all these different combinations and how we can make use of data to detect these formations over the course of the match and put it into context. For example, how a team’s formation changes or how they react to going behind or after a red card and in different phases of play.
Everyone who watches football sees the predicted formation based on the lineup - that’s just what the experts or pundits of that TV program have predicted because the teams don’t announce their formation! If you look at different sources like Sofascore or FotMob, you’ll often see that the formations have been reported differently, and of course, during the match something different might happen.
So the whole idea (of my research) is how to make use of tracking data to identify formations. Mainly for post-match reports and opposition analysis to get a better picture of exactly what happened on the pitch, of course, it can also have player recruitment applications if we focus on the positions (RB, CDM, …) players had experience playing in.
"I’m mainly focusing on studying team tactical formations from tracking data ... and how we can make use of data to detect these formations over the course of the match and put it into context"
[ Where does the data to train your models come from? ]
There are 2 main sources for the data that I'm using.
Optical tracking data - where cameras are installed around the stadium from different angles - use computer vision or object tracking methods to track players and the ball in 25 frames/second, or 10 frames/second, depending on the technology to give us the coordinates of every ‘object’ on the pitch.
The other source comes from TV broadcasts, it’s still tracking data but has a different frame rate or some players are missing. Depending on the provider, they might fill in the missing players from the TV frames but not always.
My project is really about developing a method, a solution that, independent of which match you are looking for - whether you are looking for a match in 2008 or the match right now - if you have the data, then you can identify the formations over the match in different phases, and take the context into account.
[ How do you accommodate for things like ‘in possession’ and ‘out of possession’ formations and different game states more broadly? ]
Yes, we can report the formations in and out of possession. Probably a lot of people know that a 3-5-2 in possession might look more like a 5-3-2 out of possession for example. It can be even more interesting to divide this into smaller phases - like the build-up, progression, and finishing. In the finishing stage (the last 3rd), the team usually looks much different to how their formation might predict. That’s why dividing the match into smaller time windows and knowing which windows to look at are important - you don’t want to include set pieces for example because the tracking data will skew the rest of the formation. We will have a presentation on match phases at the upcoming StatsBomb conference in Manchester.
[ I recently listened to some pundits talking about the growth of analytics in football - their consensus was that the use of analytics has had a huge impact on scouting and transfer but that in terms of the actual play on the pitch, not much has changed - do you agree? ]
I think when it comes to transfers, it’s much easier to assess the impact (of a ‘good’ or ‘bad’ transfer) because the numbers are there. So that’s why data can be used there mainly for scouting and recruitment purposes to create filters for finding the best players. I think for clubs this makes sense because they are always looking to decrease costs and increase revenue.
When it comes to the game itself, maybe if we look at the last 20 years, you would see more shots taken from long distances. Nowadays, you don’t see this happening so frequently - maybe that comes down to expected goals (xG) and teams are trying to get into areas with a better chance (xG) before shooting.
Of course, teams use data differently. One approach is using data to find out the optimal way to perform a specific action, for example, whether an inswinging or outswinging corner is best.
Another approach is closer to the game model - how the coaches want to play - and using data to monitor only their own behavior and performance. For example, they might want to find all the moments when their team is in a low block. They can use data in a supportive way to automatically find all the video clips from the last matches. This saves the time of analysts in cutting those video clips and they can focus on other areas.
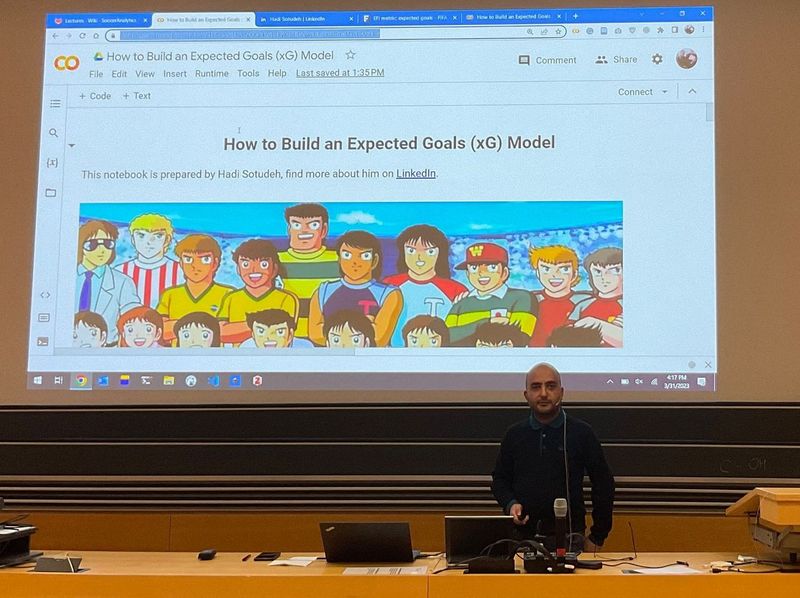
[ What is the relationship between academia and professional clubs/’the business of football’? ]
I think it depends on the club and whether they have the people - or a data science department to look at what is happening in academia. A lot of clubs don’t have any department or in-house team and are relying on 3rd party solutions. In that case, they’re just waiting for the software company to implement new things in their solution - then it becomes a question of whether what we do in academia is of relevance to those people in the software industry. Of course, we have different incentives and goals.
[ Are you trying to ‘prove’ a thesis/theory about certain tactics or formations? ]
No, no, we’re not interested in finding the ‘best formation’, that’s not the goal of my work.
The idea is that, for example, if you are a football club in Germany, and you are playing a club from Poland in the Europa League and you don't have any clue about that team, you’ll need to learn about them and how they play.
In the past, you could do this ‘manually’ by asking your team to go through the videos but if you have this data and can automatically find e.g. the last 5 matches and the exact formations in and out of possession and which players are occupying those positions - it gives you a much clearer picture of the team and can help you understand where to focus on. We are ‘assisting’ in that process.
[ What are the next advanced metrics that have the potential to go ‘mainstream’? ]
There's a metric from a company in Germany, Impect, called ‘packing’. It’s probably not that well known in the media, or at least you don’t see it often but essentially it’s trying to quantify which team had the higher chance to win the match by counting how many players are ‘outplayed’ by each pass.
—^interviewer’s note: Impect was founded by former Bayer Leverkusen teammates Stefan Reinartz and Jens Hegeler
I think that apart from these metrics, what’s probably undervalued in the football data space is the amount of publicly available data about a player’s off-pitch behavior. Some years ago, I attended a webinar on recruitment and there was someone who used to work at Scotland Yard and he is working in an intelligence company now. Clubs would come to him and ask him to investigate a specific player (a transfer target) and find out more about the player, because they want to know everything about the player before signing him.
Their social media activities for example, could start having a bigger influence on the recruitment process. You can see what kind of content they are producing or what time of day they’re posting - e.g. if you see a player posting a lot at 2am it can be an indication of something to look into. There’s a lot of data from other sources like transfermarkt with a full history of the players and their injuries or all the clubs they’ve been at, or which academies they started in. So I think, while a lot of attention has been given to on-pitch performance, this is an area that’s received less attention.
[ Do you think football metrics based on large amounts of data are skewed because of the availability of data from certain leagues/countries? ]
Some leagues, some countries have more and better data coverage than others. Usually it’s because there is a demand - there are clients who are asking for it and the data provider decides, ‘Okay it makes sense to start collecting this data’. Maybe in the 5th division, there’s no demand for data so they just don’t collect it.
What will the impact be? Because you’re using that data to train and build some models, those models are best used for the same league, or similar leagues at the same level.
What I see though, is that if you’re a data scientist who wants to work for a club in the 5th division, you’ll face problems because there’s no data! This job as a data scientist will be changed to try to find solutions that create and/or generate data - this is exactly the task that data providers are doing.
If you have to try and generate the data, it (likely) won’t be at the same standard as a data provider. There are some companies, or even publicly available solutions on Github, that are using TV broadcasts to generate tracking data but they still need a lot of fine-tuning.
[ You are quite notable on LinkedIn for posting football data jobs - why did you start sharing these? How do you find and ‘assess’ them? ]
When I graduated from the doctorate in engineering program in the Netherlands, I was looking for my next job and found it quite difficult to find job positions online. There are some websites where you can find jobs and apply but I didn’t feel they had enough options - maybe only one or two per month.
If you’re looking for a data scientist position in a bank, you go to the website of the bank, the careers section, and probably you will find something. That wasn’t the case for football clubs.
Maybe one or two years ago, I started sharing a few positions on LinkedIn. There seemed to be interest in these types of jobs. I then developed a solution to automatically collect all these football data related positions. Then I go through the descriptions and make sure the role is relevant. Sometimes I exclude some jobs, for example, I’m not interested in the betting side of football, so I don’t post any of these positions. I schedule it to go out automatically on LinkedIn, usually at the same time each day - so it’s scalable and I don’t need to do too much manually.
"If you’re looking for a data scientist position in a bank, you go to the website of the bank, the careers section, and probably you will find something. That wasn’t the case for football clubs."
[ You’re a resource for others finding jobs - how do your own post-PhD plans look? ]
I still have about one and a half years until my graduation. I’ll start looking for jobs then - probably I’ll stop sharing job postings so much because I don’t want to create more competition!
I think I’ll enter into an unemployment period for some months while I’m looking for the right opportunity. One of the difficult things in the football industry is many of the jobs are based in the UK - which makes sense because of the market they have in the Premier League - but there is an obvious visa limitation. Finding out if you have a work permit is usually part of the first screening call and removes a lot of people from the process.
From time to time, there are openings in countries like Germany, France, or Spain but of course, the language barrier is there! The US is also interesting because it’s not as close to foreigners in terms of the work permit and there are a lot of clubs starting to use data but the quality of football is not the highest. So let’s see - I would say it really depends on how the market develops when I’m looking for a job!
If you are interested in learning more about this topic, check Hadi’s FAQ post.